Penerapan Metode Bidirectional Gated Recurrent Unit dalam Identifikasi Judul Berita Clickbait Berbahasa Indonesia
The Application of Bidirectional Gated Recurrent Unit (BiGRU) Method in Identifying Clickbait News Headlines in Indonesian Language
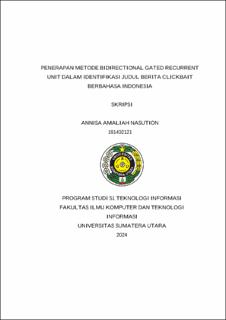
Date
2024Author
Nasution, Annisa Amaliah
Advisor(s)
Huzaifah, Ade Sarah
Hizriadi, Ainul
Metadata
Show full item recordAbstract
The increasingly advanced technology makes it easier for people to access information through online news portals. The results of a Nielsen Media Research survey show that the number of news readers on digital online media is 6 million people, exceeding the number of readers in print media, which is 4.5 million people. The surge in visitors to news portals has created fierce competition among media to attract readers as a means of maximizing revenue. News writers compete with each other to increase the number of readers, often using provocatively styled headlines to pique readers' curiosity, a strategy commonly known as Clickbait. The use of Clickbait news headlines can impact the decline in news quality and even potentially lead to misinformation. This can also be dangerous for society due to low literacy levels. As a preventive measure against Clickbait news, readers are encouraged to classify early. Therefore, it is important to develop a technique capable of classifying news headlines as Clickbait or not. This research aims to obtain a Clickbait news headline classification model using Deep Learning methods, namely Bidirectional Gated Recurrent Unit, and Fasttext word embedding to represent words into vectors. This model uses 11,279 data which is divided by 80% training data and 20% test data so that it can produce the best model with 93% performance in classifying clickbaitnews titles in Indonesian. The implementation of the Clickbait news headline classification model in the form of a system should also be considered so that it can be easily used by the public in real-time. This research utilizes the utilization of a website and a Chrome extension for system implementation.
Collections
- Undergraduate Theses [765]