Penentuan Outlier pada Data Multivariat Berbasis Jarak dengan Kuantil
Determining Outliers in Multivariate Data Based on Distance with Quantile
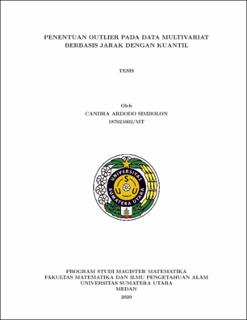
Date
2020Author
Simbolon, Candra Ardodo
Advisor(s)
Darnius, Open
Sutarman
Metadata
Show full item recordAbstract
The quantile model is a method for detecting data that is classified as outliers.
There are many methods that have been applied by researchers before. Some of
them are the minimum volume ellipsoid (MVE) method, the minimum covariance
determinant (MCD) method, the data-mining method. This method is considered
less efficient in detecting outliers for multivariate data so it needs to be further
developed with another method which is considered more efficient and more ac-
curate. Outlier cases in this research are focused on multivariate data which
has been transformed into univariate data (1 dimension). Next, the average and
median are taken as data centers so that the distance between each data can be
calculated. The concept of distance is also important in carrying out calculations
in this discussion. Distance calculations use Mahalanobis and Euclid’s equations.
Next, it will be detected using the quantile model after meeting the criteria that
have been set as a reference for data that is said to be classified as an outlier,
namely based on the 90% quantile and 95% quantile values.
Collections
- Master Theses [412]