Implementasi Model Machine Learning Algoritma Regresi Linier dan Regresi Logistik pada Prediksi Hasil Pertandingan Sepak Bola Liga 1 Indonesia 2024/2025
Implementation of Machine Learning Models of Linear Regression and Logistic Regression Algorithms in Predicting the Results of the 2024/2025 Indonesian League 1 Football Matches
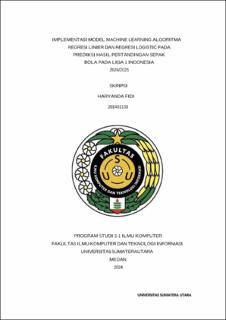
Date
2025Author
Fidi, Haryanda
Advisor(s)
Zamzami, Elviawaty Muisa
Handrizal
Metadata
Show full item recordAbstract
Football is the most popular sport globally, including in Indonesia. Liga 1
Indonesia, as the country’s top-tier football competition, garners the attention of
millions of fans and industry stakeholders. However, predicting football match
outcomes is a complex challenge involving various factors such as team
performance, player statistics, and match conditions. This study aims to develop
a predictive model for match outcomes in the 2024/2025 Liga 1 Indonesia
season by implementing linear regression and logistic regression algorithms.
The methodology involves collecting historical match data, including scores,
wins, losses, draws, as well as other variables like the number of shots, ball
possession, and individual player statistics. The data undergoes cleaning,
normalization, and feature analysis to ensure quality. A linear regression model
is used to predict match scores, while a logistic regression model is employed to
classify match outcomes into win, loss, or draw categories. Model performance
is evaluated using metrics such as Mean Squared Error (MSE) for linear
regression and accuracy, precision, recall, and F1-score for logistic regression.
The results show that the linear regression model predicts match scores with an
MSE of 0.75, while the logistic regression model achieves an outcome prediction
accuracy of 82%. These findings indicate that the combination of these two
algorithms is effective for predicting Liga 1 Indonesia football match outcomes.
The study concludes that machine learning-based models, particularly linear and
logistic regression algorithms, can serve as reliable tools for match analysis,
providing strategic insights for coaches and enhancing fan experiences through
more accurate predictions.
Collections
- Undergraduate Theses [1235]