Optimisasi Metode Klasifikasi KNN, SVM, dan SVM Kernel pada Prediksi Potabilitas Air dengan Pendekatan Hyperparameter
Optimization of KNN, SVM, and SVM Kernel in Water Potability Prediction with Hyperparameter Approach
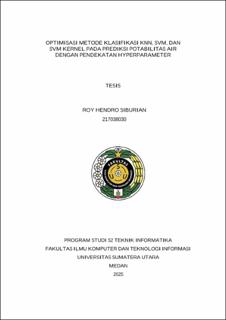
Date
2025Author
Siburian, Roy Hendro
Advisor(s)
Mahyuddin
Tarigan, Jos Timanta
Metadata
Show full item recordAbstract
his research focuses on optimizing the K-Nearest Neighbor (KNN), Support
Vector Machine (SVM), and SVM Kernel classification methods using a
hyperparameter approach to improve the accuracy of water potability prediction.
The water potability dataset containing 3276 water samples with 10 features is
used. The research methods include exploratory data analysis (EDA), data
preprocessing (handling missing values, standardization, dimensionality reduction,
and feature selection), modeling (KNN, SVM, SVM Kernel), hyperparameter
optimization using GridSearchCV, and model evaluation (accuracy, precision,
recall, F1-score, ROC AUC). The results show that the SVM model with an
optimized RBF kernel has the best performance, but the overall model accuracy is
still not optimal. Further research is suggested to address class imbalance, select
more relevant features, engineer features, and use ensemble techniques. With
further development, it is expected that a more accurate and reliable model for
predicting water potability can be produced, thereby contributing to improving
public health and well-being.
Collections
- Master Theses [620]