Analisis dan Optimalisasi Penempatan Electric Vehicle Charging Station dengan Hybrid Particle Swarm Optimization dan Genetic Algorithm (Studi Kasus PT PLN UP3 Medan Kota)
Analysis and Optimization of Electric Vehicle Charging Station Placement with Hybrid Particle Swarm Optimization and Genetic Algorithm (Case Study of PT PLN UP3 Medan Kota)
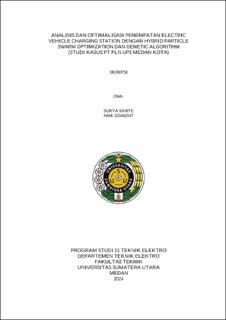
Date
2024Author
Sihite, Surya
Advisor(s)
Mubarakah, Naemah
Bukit, Ferry Rahmat Astianta
Metadata
Show full item recordAbstract
The adoption rate of electric vehicles (EVs) in the transportation sector is closely linked to the growing interest in environmentally friendly transportation initiatives. However, ineffective placement of Electric Vehicle Charging Stations (EVCS) can lead to technical challenges and energy wastage within the distribution system. It is crucial to strategically position EVCS to mitigate the impact on the electric vehicle load. In this study, we employ a Hybrid Particle Swarm Optimization – Genetic Algorithm (HPSO-GA) to optimize the placement of EVCS. The analysis focuses on calculating power flow within the load. The primary objective of this investigation is to minimize power losses and enhance voltage profiles within the system. The proposed approach is tested on the LK 10 UP3 Medan Kota feeder and compared with particle swarm optimization (PSO) and genetic algorithm (GA) techniques. The simulation results for scenario 1 produced a power loss of 310.9 KW and 227.6 KVar. Scenario 2 resulted in a power loss of 368.3 KW and 269.5 KVar. Scenario 3, using HPSO-GA, produced a power loss of 316.3 KW and 231.5 KVar. Scenario 4 resulted in a power loss of 445.2 KW and 325.8 KVar, and scenario 5, using HPSO-GA, produced a power loss of 331.3 KW and 242.5 KVar. Simulation results demonstrate the effectiveness of HPSO-GA, indicating that integrating 3 EVCS with 5 EVCS yields optimal outcomes in the network compared to manually placing EVCS at specific locations. This integration results in a power loss reduction of 14.10% for 3 EVCS buses and 25.55% for 5 EVCS. Overall, the proposed approach enhances system performance across all metrics, highlighting the superior performance of HPSO-GA compared to PSO and GA in achieving the specified goals.
Collections
- Undergraduate Theses [1401]