Integrasi Model Deep Learning dengan Pre-trained MobileNetV2 dan Speech Recognition pada Aplikasi Video Call ElCue untuk Pendeteksian Bahasa Isyarat Indonesia (BISINDO)
Integration of Deep Learning Model with Pre-trained MobileNetV2 and Speech Recognition in ElCue Video Call Application for Detection of Indonesian Sign Language (BISINDO)
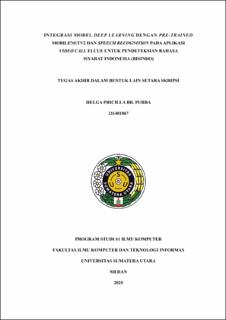
Date
2025Author
Purba, Helga Pricilla Br.
Advisor(s)
Hayatunnufus, Hayatunnufus
Lydia, Maya Silvi
Metadata
Show full item recordAbstract
This research focuses on the integration of the MobileNetV2 deep learning model and Speech Recognition technology in a video call application to detect Indonesian Sign Language (BISINDO) gestures and convert speech-to-text in real-time. The main objective of this study is to facilitate more inclusive two-way communication between Deaf and non-Deaf users in the context of video calls. The ElCue application integrates both technologies, with MobileNetV2 used to detect the hand gestures of Deaf users and translate them into text, while Speech Recognition is used to transcribe the speech of non-Deaf users into text. The MobileNetV2 model, converted into TensorFlow Lite (TFLite) format, successfully detected BISINDO gestures with an average accuracy of 85.56%. Meanwhile, Speech Recognition technology, through the integration of the Speech-to-Text API, transcribed speech with an average accuracy of 93%. The use of Agora SDK for video calls ensures smooth audio and video communication, despite the real-time processing requirements of AI technologies. Testing results show that this integration successfully enhances communication accessibility between Deaf and non-Deaf users, although challenges remain regarding the limited computational power of mobile devices. This study makes a significant contribution to the development of more inclusive communication technologies for the Deaf community.
Collections
- Undergraduate Theses [1171]