Lexicon Based Data Labelling untuk Peningkatan Kinerja Long Short-Term Memory dalam Analisis Sentimen
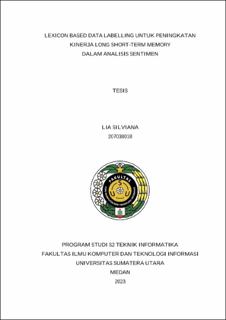
Date
2023Author
Silviana, Lia
Advisor(s)
Nababan, Erna Budhiarti
Zarlis, Muhammad
Metadata
Show full item recordAbstract
Accuracy in training sentiment analysis models for large number of review datasets is affected by the correct classification of sentiment labels. Improving the accuracy of sentiment labels, text representation also affects the performance of sentiment analysis models. Deep learning methods have been widely used to solve various sentiment analysis problems. To improve the performance of deep learning in sentiment analysis, it is necessary to use the right labeling method and good text representation to be used as an embedding layer. This study proposes sentiment labeling using Lexicon and deep learning Long Short-Term Memory (LSTM) and FastText as embedding words in sentiment classification. The InSet Lexicon Dictionary is used as a corpus in performing feature extraction. The sentiment data used is the reviews given by users on several applications provided on Google Play. The results showed that the LSTM network using Word embedded FastText with a dimension of 300 words received a small error value of 0.111 with an accuracy of 95.55% for data labeled based on Lexicon.
Collections
- Master Theses [620]