Peningkatan Akurasi Long Short-Term Memory (LSTM) Menggunakan Fasttext dan Glove untuk Penerjemah Bahasa Batak-Indonesia
Improving The Accuracy of Long Short Term Memory (LSTM) Using Fasttext and Glove for Batak - Indonesian Translator
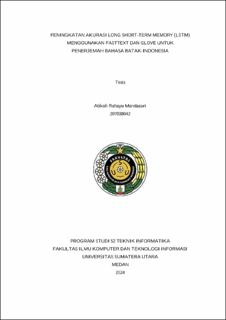
Date
2024Author
Mandasari, Atikah Rahayu
Advisor(s)
Nababan, Erna Budhiarti
Mawengkang, Herman
Metadata
Show full item recordAbstract
Automatic translation of regional languages into Indonesian is a complex challenge in
the field of natural language processing (NLP). This research aims to improve the
accuracy of the Long Short Term Memory (LSTM) model in translating Batak language
to Indonesian by leveraging two popular word embedding techniques, FastText and
Glove. LSTM is chosen for its capability in handling long and complex sequences of
data. FastText is used to capture richer word representations by considering sub-word
information, while Glove is employed to obtain more global word representations based
on the context in large corpora. Experiments are conducted by combining embeddings
from FastText and Glove into the LSTM architecture. Results show that this
combination can improve translation accuracy compared to the individual use of
embeddings. Evaluation is performed using the BLEU metric to measure translation
quality. This research contributes to the development of more effective regional
language translation systems and demonstrates the potential of combining embedding
techniques in LSTM models for other NLP applications.
Collections
- Master Theses [620]