Perbandingan Klasifikasi Jenis Citra Awan Menggunakan Algoritma YOLO V8 dan Faster R-CNN
Comparison of Cloud Image Type Classification Using YOLO V8 and Faster R-CNN Algorithm
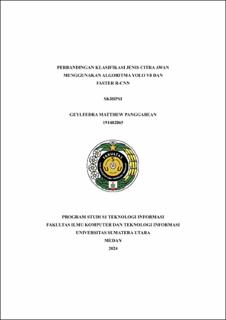
Date
2024Author
Panggabean, Geylfedra Matthew
Advisor(s)
Rahmat, Romi Fadillah
Lubis, Fahrurrozi
Metadata
Show full item recordAbstract
Cloud observation and analysis play a crucial role in many aspects of daily life, including weather prediction. Along with the development of technology, cloud image classification has become a focus of research to support these applications. However, not many studies have used approaches such as Faster R-CNN and YOLO v8 in cloud image classification. This study aims to compare the effectiveness of cloud image classification using YOLO v8 and Faster R-CNN algorithms. The data used comes from the Singapore Whole sky Imaging CATegories Database (SWIMCAT) and includes five cloud categories: clear sky, pattern cloud, thick dark cloud, thick cloud, and veil. Through a series of experiments and tests, the results show that the YOLO v8 algorithm, especially with the pre-trained model yolov8m-cls, provides better accuracy than Faster R-CNN in classifying cloud images, with accuracy reaching 97.43% when tested whereas the model when using Faster R-CNN algorithm reached the accuracy of 64.10%. However, the study also highlighted that failures in classification can occur due to similar cloud characteristics, which can be affected by the time of image capture. In conclusion, cloud image classification using YOLO v8 showed better performance, but understanding cloud characteristics and managing image capture time remain key factors in the success of this application.
Collections
- Undergraduate Theses [765]