Perbandingan Prediksi Radiasi Sinar Matahari Menggunakan Algoritma Gated Recurrent Unit (GRU), Long Short-Term Memory (LSTM) dan Deep Q-Network (DQN)
Prediction of Solar Radiation Using Hybridization of Deep Q-Network (DQN) and Gated Recurrent Unit (GRU) Algorithms
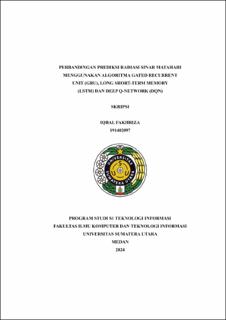
Date
2024Author
Fakhriza, Iqbal
Advisor(s)
Rahmat, Romi Fadillah
Purnamawati, Sarah
Metadata
Show full item recordAbstract
Long-term prediction of solar radiation is very important in providing detailed information about solar resources in a region. For entrepreneurs, investors and governments, this is very useful for large-scale solar energy development. The information allows them to make better decisions regarding solar energy investments and policies in a region. Several previous studies have predicted solar radiation with various methods ranging from using physical models such as Numerical Weather Prediction (NWP), statistical models, to using artificial intelligence models. In this research, 3 algorithms will be compared to define the best algorithm in solar radiation prediction object. These algorithms are Gated Recurrent Unit (GRU), Long Short-Term Memory (LSTM) and Deep Q-Network (DQN). The data that will be used in this research comes from the results of the research Development of software for estimating clear sky solar radiation in Indonesia by Himsar Ambarita (2017) with data coverage for 7 years in total. Later as a model evaluation, 4 evaluation metrics will be used to calculate the amount of model error. These evaluation metrics include Mean Squared Error (MSE), Root Mean Squared Error (RMSE), Mean Absolute Error (MAE), and R2 (Coefficient of Determination). The main objective of this research is to compare the accuracy of regression algorithms such as GRU and LSTM compared to the DQN algorithm which has a calculation based on reward values in predicting solar radiation.
Collections
- Undergraduate Theses [765]