Optimasi Kombinasi Parameter Cost dan Kernel SVM melalui Gridsearch
SVM Cost and Kernel Parameter Combination Optimization by GridSearch
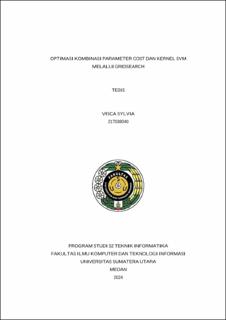
Date
2024Author
Sylvia, Visca
Advisor(s)
Lydia, Maya Silvi
Nasution, Benny Benyamin
Metadata
Show full item recordAbstract
The aim of this research is to improve the performance of the Support Vector Machine (SVM) algorithm in determining the values of Cost and Kernel parameters using the GridSearch technique. This study focuses on sentiment analysis of trainee reviews at the Balai Besar Pelatihan Vokasi dan Produktivitas (BBPVP) Medan. Reviews were collected and analyzed to assess the effectiveness of the training programs. The methodology includes data preprocessing, text transformation using TF-IDF, and the application of SVM optimized with GridSearch. The results show that using GridSearch for SVM parameter optimization significantly enhances performance compared to the non-optimized SVM model. Overall accuracy, precision, recall, and F1-Score improved, indicating that the optimized model is more effective in classifying the sentiments of trainee reviews. This research makes a significant contribution to the application of machine learning methods for sentiment analysis, particularly in the context of evaluating job training programs.
Collections
- Master Theses [623]