Algoritma Hibrid Menggunakan CNN dan LSTM untuk Peningkatan Performansi pada Klasifikasi Citra Lesi Kulit
Hybrid Algorithm Using CNN and LSTM to Improveperformance in Skin Lesion Image Classification
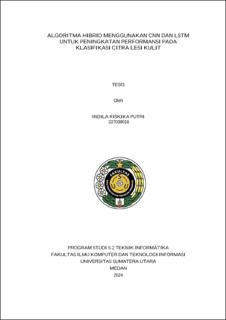
Date
2024Author
Putri, Indila Riskika
Advisor(s)
Amalia
Abidin, Taufik Fuadi
Metadata
Show full item recordAbstract
Skin lesions areacondition of abnormal growths or changes in theappearanceofthe skin, such as lumps, abrasions, cysts, or colordifferences. Skin lesionscan bedetected as cancerous and non-cancerous. Detecting earlier skin lesions can beachieved through variousmethods, including the useof dermoscopic tools with the assistanceof adermatologist. Dermoscopy has enhanced accuracy in diagnosing the early stages of skin cancer.However,the persistent challengeof relatively lowaccuracy rates stilloccurs. Although adermatoscopy is used, the identification of skin cancer remains imperfect. Artificial Intelligence(AI) has emergedas apotential solution to address this issueof skin lesions image-based diagnoses. This research proposes adeep learning approach employing ahybrid algorithm that integrates Convolutional Neural Networks (CNN) with Long Short-Term Memory(LSTM) networks. This method aims toenhancethe efficacy of early skin lesiondetection and classification. Thedataset employed in thisstudycomprised 2,736imagesamples from acombination of twodatasets, PAD-UFES-20 and ISICdataset. Thesesamples arecategorizedinto six distinct classes: Actinic Keratosis(ACK), Basal CellCarcinoma(BCC), Malignant Melanoma(MEL), MelanocyticNevus (NEV), SquamousCellCarcinoma(SCC), and Seborrheic Keratosis(SEK). Theimages wereadjusted to 300 x300 pixels, and data augmentation techniqueswereapplied to perform new data. Theproposed method utilizes CNNforimagefeatureextraction and LSTM forlearning and classification. Theproposed networkconsistsof fivelayers of convolution, fivepoolinglayers, and onelayer forLSTM.Theresearch findings show an improvement in accuracy, increasing from96.27%with the CNNnetworkto 97.26%after integrating the CNN-LSTM network. Inaddition, the integratedCNN-LSTM networkmodel demonstrated 91.83%sensitivity, 91.83%precision,and 91.82%F1-Score. This finding surpassed theperformanceof the CNN network, which achieved lower metrics with 88.83%sensitivity, 88.83%precision, and 88.82%F1-Score. This research contributes to the early detection of skin diseases through featureextraction techniques, which canbethe basisfordeveloping amoreaccurateandbetter skin lesion classification system.
Collections
- Master Theses [620]